Open Access
ARTICLE
ResNet CNN with LSTM Based Tamil Text Detection from Video Frames
1 Department of Electronics & Communication Engineering, Government College of Engineering, Thanjavur, 613402, India
2 Department of Electronics & Communication Engineering, Mahendra College of Engineering, Salem, 636106, India
3 Department of Computer Science & Engineering, AC Govt. College of Engineering & Technology, Karaikudi, 630004, India
* Corresponding Author: I. Muthumani. Email:
Intelligent Automation & Soft Computing 2022, 31(2), 917-928. https://doi.org/10.32604/iasc.2022.018030
Received 22 February 2021; Accepted 03 June 2021; Issue published 22 September 2021
Abstract
Text content in videos includes applications such as library video retrievals, live-streaming advertisements, opinion mining, and video synthesis. The key components of such systems include video text detection and acknowledgments. This paper provides a framework to detect and accept text video frames, aiming specifically at the cursive script of Tamil text. The model consists of a text detector, script identifier, and text recognizer. The identification in video frames of textual regions is performed using deep neural networks as object detectors. Textual script content is associated with convolutional neural networks (CNNs) and recognized by combining ResNet CNNs with long short-term memory (LSTM) networks. A residual mapping underlies the network. In ResNet, skipping condenses the network into few layers to help it learn more quickly. Bidirectional LSTM enables networks to always have information about the sequence, backward as well as forward. For fast pattern learning processes, this combination uses the residual learning process. In comparison to the existing approaches, the proposed approach’s results show improved accuracy, precision and F-measures. The combination of ResNet CNNs and bidirectional LSTMs has high recognition rates for detecting video texts in Tamil cursive script.Keywords
Cite This Article
Citations
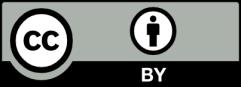
This work is licensed under a Creative Commons Attribution 4.0 International License , which permits unrestricted use, distribution, and reproduction in any medium, provided the original work is properly cited.