Open Access
ARTICLE
Enrichment of Crop Yield Prophecy Using Machine Learning Algorithms
Department of Computer Science and Engineering, Sri Ramakrishna Engineering College, Coimbatore, 641653, India
* Corresponding Author: R. Kingsy Grace. Email:
Intelligent Automation & Soft Computing 2022, 31(1), 279-296. https://doi.org/10.32604/iasc.2022.019947
Received 01 May 2021; Accepted 02 June 2021; Issue published 03 September 2021
Abstract
Strong associations exist between the crop productivity and the seasonal, biological, economical causes in natural ecosystems. The linkages like climatic conditions, health of a soil, growth of crop, irrigation, fertilizers, temperature, rainwater, pesticides desired to be preserved in comprehensively managed crop lands which impacts the crop potency. Crop yield prognosis plays a vibrant part in agricultural planning, administration and environs sustainability. Advancements in the field of Machine Learning have perceived novel expectations to improve the prediction performance in Agriculture. Highly gratifying prediction of crop yield helps the majority of agronomists for their rapid decision-making in the choice of crop to be cultivated. This paper makes an attempt to suggest which crop can be sown at a particular district in Tamil Nadu, depending on the factors required for the growth of the crop based on the research outcomes. This is achieved by applying clustering on the attributes in the dataset using E-DBSCAN and is compared with three different algorithms such as DBSCAN, CLARA and K-means. The best suitable factor for the growth of a crop for a location is predicted using clustering techniques. The accuracy of crop yield prediction is calculated for three crops, namely, rice, wheat and maize. The proposed method outperforms in terms of Bias, F1 Score, MAPE, MDAE, MSE, RAE, RMSE and MAE with existing algorithms in the literature.Keywords
Cite This Article
Citations
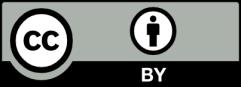
This work is licensed under a Creative Commons Attribution 4.0 International License , which permits unrestricted use, distribution, and reproduction in any medium, provided the original work is properly cited.