Open Access
ARTICLE
Energy Demand Forecasting Using Fused Machine Learning Approaches
1 Center for Cyber Security, Faculty of Information Science and Technology, Universiti Kebansaan Malaysia (UKM), Bangi, 43600, Selangor, Malaysia
2 School of Information Technology, Skyline University College, University City Sharjah, Sharjah, 1797, UAE
3 School of Computer Science, NCBA&E, Lahore, 54000, Pakistan
4 Canadian University Dubai, Dubai, UAE
5 Pattern Recognition and Machine Learning Lab, Department of Software, Gachon University, Seongnam, 13557, Korea
6 School of Computer Science, Minhaj University Lahore, Lahore, 54000, Pakistan
* Corresponding Author: Muhammad Adnan Khan. Email:
Intelligent Automation & Soft Computing 2022, 31(1), 539-553. https://doi.org/10.32604/iasc.2022.019658
Received 21 April 2021; Accepted 04 June 2021; Issue published 03 September 2021
Abstract
The usage of IoT-based smart meter in electric power consumption shows a significant role in helping the users to manage and control their electric power consumption. It produces smooth communication to build equitable electric power distribution for users and improved management of the entire electric system for providers. Machine learning predicting algorithms have been worked to apply the electric efficiency and response of progressive energy creation, transmission, and consumption. In the proposed model, an IoT-based smart meter uses a support vector machine and deep extreme machine learning techniques for professional energy management. A deep extreme machine learning approach applied to feature-based data provided a better result. Lastly, decision-based fusion applied to both datasets to predict power consumption through smart meters and get better results than previous techniques. The established model smart meter with automatic load control increases the effectiveness of energy management. The proposed EDF-FMLA model achieved 90.70 accuracy for predicting energy consumption with a smart meter which is better than the existing approaches.Keywords
Cite This Article
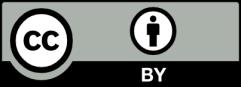
This work is licensed under a Creative Commons Attribution 4.0 International License , which permits unrestricted use, distribution, and reproduction in any medium, provided the original work is properly cited.