Open Access
ARTICLE
Deep Learning Based Stacked Sparse Autoencoder for PAPR Reduction in OFDM Systems
1 Department of Electronics and Communication Engineering, K.S.R. College of Engineering, Tiruchengode, 637215, India
2 Department of Electronics and Communication Engineering, University College of Engineering, BIT Campus, Anna University, Tiruchirappalli, 620024, India
* Corresponding Author: T. Jayasankar. Email:
Intelligent Automation & Soft Computing 2022, 31(1), 311-324. https://doi.org/10.32604/iasc.2022.019473
Received 14 April 2021; Accepted 20 May 2021; Issue published 03 September 2021
Abstract
Orthogonal frequency division multiplexing is one of the efficient and flexible modulation techniques, and which is considered as the central part of many wired and wireless standards. Orthogonal frequency division multiplexing (OFDM) and multiple-input multiple-output (MIMO) achieves maximum spectral efficiency and data rates for wireless mobile communication systems. Though it offers better quality of services, high peak-to-average power ratio (PAPR) is the major issue that needs to be resolved in the MIMO-OFDM system. Earlier studies have addressed the high PAPR of OFDM system using clipping, coding, selected mapping, tone injection, peak windowing, etc. Recently, deep learning (DL) models have exhibited improved performance on channel estimation, signal recognition, channel decoding, modulation identification, and end-to-end wireless system. In this view, this paper presents a new Hyperparameter Tuned Deep Learning based Stacked Sparse Autoencoder (HPT-SSAE) for PAPR Reduction Technique in OFDM system. The proposed model aims to substantially reduce the peaks in the OFDM signal. The presented HPT-SSAE model is utilized to adaptively create a peak-canceling signal based on the features of the input signal. In the HPT-SSAE model, the constellation mapping and demapping of symbols take place on every individual subcarrier adaptively using the SSAE model in such a way that bit error rate (BER) and the PAPR of the OFDM systems are cooperatively diminished. Besides, to enhance the performance of the SSAE model, the hyperparameter tuning process takes place using monarch butterfly optimization (MBO) algorithm. A comprehensive set of simulations were performed to highlight the supremacy of the HPT-SSAE model. The obtained experimental values showcased the betterment of the proposed model over the compared methods interms of bit error rate (BER), complementary cumulative distribution function (CCDF), and execution time.Keywords
Cite This Article
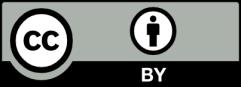
This work is licensed under a Creative Commons Attribution 4.0 International License , which permits unrestricted use, distribution, and reproduction in any medium, provided the original work is properly cited.