Open Access
ARTICLE
Deep Learning-Based Skin Lesion Diagnosis Model Using Dermoscopic Images
1 Department of Information Technology, P. V. P. Siddhartha Institute of Technology, Vijayawada, 520007, India
2 Department of Education Counselling, College of Basic Education University of Duhok, Duhok, 44001, Iraq
3 Department of Computer Science & Engineering, South Point Group of Institutions, Sonipat, Haryana, 131001, India
4 Department of ECE, Saveetha School of Engineering, SIMATS, Saveetha University, Tamil Nadu, 602105, India
5 Department of CSE, Sree Vidyanikethan Engineering College, Tirupati, 517102, India
6 Department of Information Technology, College of Engineering, Catholic University in Erbil, Kurdistan Region, 44001, Iraq
7 Department of Information Technology, Jeppiaar Institute of Technology, 601201, India
* Corresponding Author: G. Reshma. Email:
(This article belongs to the Special Issue: Intelligence 4.0: Concepts and Advances in Computational Intelligence)
Intelligent Automation & Soft Computing 2022, 31(1), 621-634. https://doi.org/10.32604/iasc.2022.019117
Received 02 April 2021; Accepted 11 June 2021; Issue published 03 September 2021
Abstract
In recent years, intelligent automation in the healthcare sector becomes more familiar due to the integration of artificial intelligence (AI) techniques. Intelligent healthcare systems assist in making better decisions, which further enable the patient to provide improved medical services. At the same time, skin lesion is a deadly disease that affects people of all age groups. Skin lesion segmentation and classification play a vital part in the earlier and precise skin cancer diagnosis by intelligent systems. However, the automated diagnosis of skin lesions in dermoscopic images is challenging because of the problems such as artifacts (hair, gel bubble, ruler marker), blurry boundary, poor contrast, and variable sizes and shapes of the lesion images. This study develops intelligent multilevel thresholding with deep learning (IMLT-DL) based skin lesion segmentation and classification model using dermoscopic images to address these problems. Primarily, the presented IMLT-DL model incorporates the Top hat filtering and inpainting technique for the pre-processing of the dermoscopic images. In addition, the Mayfly Optimization (MFO) with multilevel Kapur’s thresholding-based segmentation process is involved in determining the infected regions. Besides, an Inception v3 based feature extractor is applied to derive a valuable set of feature vectors. Finally, the classification process is carried out using a gradient boosting tree (GBT) model. The presented model’s performance takes place against the International Skin Imaging Collaboration (ISIC) dataset, and the experimental outcomes are inspected in different evaluation measures. The resultant experimental values ensure that the proposed IMLT-DL model outperforms the existing methods by achieving higher accuracy of 0.992.Keywords
Cite This Article
Citations
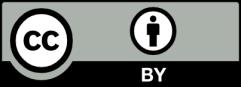
This work is licensed under a Creative Commons Attribution 4.0 International License , which permits unrestricted use, distribution, and reproduction in any medium, provided the original work is properly cited.