Open Access
ARTICLE
Bayesian Approximation Techniques for the Generalized Inverted Exponential Distribution
Department of Statistics, College of Science, University of Jeddah, Jeddah, Saudi Arabia
* Corresponding Author: Maha A. Aldahlan. Email:
Intelligent Automation & Soft Computing 2022, 31(1), 129-142. https://doi.org/10.32604/iasc.2022.018041
Received 22 February 2021; Accepted 02 May 2021; Issue published 03 September 2021
Abstract
In this article, Bayesian techniques are adopted to estimate the shape parameter of the generalized inverted exponential distribution (GIED) in the case of complete samples. Normal approximation, Lindley’s approximation, and Tierney and Kadane’s approximation are used for deriving Bayesian estimators. Different informative priors are considered, such as Jeffrey’s prior, Quasi prior, modified Jeffrey’s prior, and the extension of Jeffrey’s prior. Non-informative priors are also used, including Gamma prior, Pareto prior, and inverse Levy prior. The Bayesian estimators are derived under the quadratic loss function. Monte Carlo simulations are carried out to make a comparison among estimators based on the mean square error of the estimates. All estimators using normal, Lindley’s, and Tierney and Kadane’s approximation techniques perform consistently since the MSE decreases as the sample size increases. For large samples, estimators based on non-informative priors using normal approximation are usually better than the ones using Lindley’s approximation. Two real data sets in reliability and medicine are applling to the GIED distribution to assess its flexibility. By comparing the estimation results with other generalized models, we prove that estimating this model using Bayesian approximation techniques gives good results for investigating estimation problems. The models compared in this research are generalized inverse Weibull distribution (GIWD), inverse Weibull distribution (IWD), and inverse exponential distribution (IED).Keywords
Cite This Article
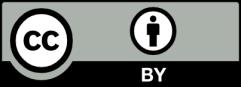