Open Access
ARTICLE
Performance Comparison of PoseNet Models on an AIoT Edge Device
1 Department of IT Transmedia Contents, Hanshin University, Osan-si, 18101, Korea
2 Hancom With Inc., Seongnam-si, 13493, Korea
* Corresponding Author: Jeongwook Seo. Email:
Intelligent Automation & Soft Computing 2021, 30(3), 743-753. https://doi.org/10.32604/iasc.2021.019329
Received 09 April 2021; Accepted 10 May 2021; Issue published 20 August 2021
Abstract
In this paper, we present an oneM2M-compliant system including an artificial intelligence of things (AIoT) edge device whose principal function is to estimate human poses by using two PoseNet models built on MobileNet v1 and ResNet-50 backbone architectures. Although MobileNet v1 is generally known to be much faster but less accurate than ResNet50, it is necessary to analyze the performances of whole PoseNet models carefully and select one of them suitable for the AIoT edge device. For this reason, we first investigate the computational complexity of the models about their neural network layers and parameters and then compare their performances in terms of inference time, frame rate and pose score. We found that the model with MobileNet v1 could estimate human poses very fast without significant pose score degradation when compared with the model with ResNet-50 through some experimental results. In addition, the model with MobileNet v1 could smoothly handle video clips with 480 × 360 and 640 × 480 resolutions as inputs except 1280 × 720 resolution. Finally, we implemented the overall oneM2M-compliant system with an AIoT edge device containing the model with MobileNet v1 and a floating population monitoring server, which is applicable to commercial area analysis in a smart city and we verified its operation and feasibility by periodically displaying all the data of temperature, humidity, illuminance, and floating population estimation from the AIoT edge device on a map in a web browser.Keywords
Cite This Article
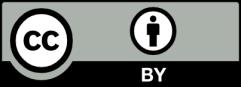
This work is licensed under a Creative Commons Attribution 4.0 International License , which permits unrestricted use, distribution, and reproduction in any medium, provided the original work is properly cited.