Open Access
ARTICLE
A Two-Step Approach for Improving Sentiment Classification Accuracy
1 Department of Computer Science & Information Technology, The Superior College Lahore, Lahore, 54000, Pakistan
2 Department of Computer Science, The University of Lahore, Lahore, 54000, Pakistan
3 Department of Electrical Engineering, Government College University, Lahore, 54000, Pakistan
4 Department of Electrical Engineering, The Superior College Lahore, Lahore, 54000, Pakistan
* Corresponding Author: Rao Muhammad Asif. Email:
Intelligent Automation & Soft Computing 2021, 30(3), 853-867. https://doi.org/10.32604/iasc.2021.019101
Received 02 April 2021; Accepted 17 May 2021; Issue published 20 August 2021
Abstract
Sentiment analysis is a method for assessing an individual’s thought, opinion, feeling, mentality, and conviction about a specific subject on indicated theme, idea, or product. The point could be a business association, a news article, a research paper, or an online item, etc. Opinions are generally divided into three groups of positive, negative, and unbiased. The way toward investigating different opinions and gathering them in every one of these categories is known as Sentiment Analysis. The enormously growing sentiment data on the web especially social media can be a big source of information. The processing of this unstructured data is of deep interest for researchers in the field of data science. The proposed article is an effort to find a model which can improve the classification accuracy of sentiment data. In this paper, a complete model is introduced to improve the accuracy of binary-class sentiment data. The model is reliable as it has been validated on three distinctive datasets with various sample sizes. In this regard, Amazon reviews, Yelp reviews, and IMDB reviews are taken into account. This model is based on completely referenced datasets and considerable improvement in accuracy is seen as compared to individual classifiers. Various heterogeneous classifiers are trained on the above-mentioned datasets at the base level. These base-level classifiers ought to be chosen from various groups of classifiers. At that point, the output of these base-level classifiers is consolidated to create a new dataset that acts as input for meta-level classifiers. At the meta-level, the base-level classifier with the best outcomes is selected with the new dataset framed. The model accordingly framed can be used to predict any sample query.Keywords
Cite This Article
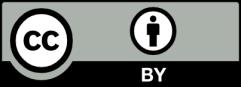
This work is licensed under a Creative Commons Attribution 4.0 International License , which permits unrestricted use, distribution, and reproduction in any medium, provided the original work is properly cited.