Open Access
ARTICLE
Decision Making Based on Fuzzy Soft Sets and Its Application in COVID-19
1 Department of Mathematics, College of Science, University of Jeddah, Jeddah, Saudi Arabia
2 Department of Mathematics, College of Science and Arts, Najran University, Najran, 66445, Saudi Arabia
3 Department of Mathematics and Statistics, College of Science, Taif University, P.O. Box 11099, Taif 21944, Saudi Arabia
* Corresponding Author: M. A. El Safty. Email:
(This article belongs to the Special Issue: Soft Computing Technologies for COVID 19 Assessment, Analysis and Control)
Intelligent Automation & Soft Computing 2021, 30(3), 961-972. https://doi.org/10.32604/iasc.2021.018242
Received 02 March 2021; Accepted 08 April 2021; Issue published 20 August 2021
Abstract
Real-world applications are now dealing with a huge amount of data, especially in the area of high-dimensional features. Trait reduction is one of the major steps in decision making problems. It refers to the determination of a minimum subset of attributes which preserves the final decision based on the entire set of attributes. Unfortunately, most of the current features are irrelevant or redundant, which makes these systems unreliable and imprecise. This paper proposes a new paradigm based on fuzzy soft relationship and level fuzzy soft relationship, called Union - Intersection decision making method. Using these new principles, the decision-making strategy is structured to choose a fuzzy set of optimal elements from the alternatives on the basis of a fuzzy soft set. Finally, we used our proposed method in medical application to make the decision to diagnose COVID-19. Moreover, we used MATLAB programming to obtain the results; this has coincided with the announcement by the World Health Organization and an accurate proposal was examined, which competes with that of the method of Zhao.Keywords
Cite This Article
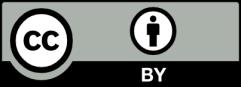