Open Access
ARTICLE
Face Image Compression and Reconstruction Based on Improved PCA
1 School of Computer and Software, Nanjing University of Information Science and Technology, Nanjing, 210044, China
2 Jiangsu Key Laboratory of Data Science and Smart Software, Jinling Institute of Technology, Nanjing, 211169, China
3 College of Information Engineering, Fuyang Normal University, Fuyang, 236041, China
4 Department of Mathematics, Faculty of Science, New Valley University, El-Kharga, 72511, Egypt
* Corresponding Author: Yu Xue. Email:
Intelligent Automation & Soft Computing 2021, 30(3), 973-982. https://doi.org/10.32604/iasc.2021.017607
Received 04 February 2021; Accepted 02 July 2021; Issue published 20 August 2021
Abstract
Face recognition technology has many usages in the real-world applications, and it has generated extensive interest in recent years. However, the amount of data in a digital image is growing explosively, taking up a lot of storage and transmission resources. There is a lot of redundancy in an image data representation. Thus, image compression has become a hot topic. The principal component analysis (PCA) can effectively remove the correlation of an image and condense the image information into a characteristic image with several main components. At the same time, it can restore different data images according to their principal components and meet the needs of image compression and reconstruction at diverse levels. This paper introduces an improved PCA algorithms. The covariance matrix, calculated according to a batch of training samples, is an approximation of the real covariance matrix. The matrix is relatively to the dimension of the covariance matrix, and the number of training samples is often too small. Therefore, it difficult to accurately obtain the covariance matrix. This improved PCA algorithm called 2DPCA can solve this problem effectively. By comparing it with several discrete PCA improvement algorithms, we show that the 2DPCA has a better dimensionality reduction effect. Compared with the PCA algorithm, the 2DPCA has a lower root-mean-square error under the constant noise condition.Keywords
Cite This Article
Y. Xue, C. Chen, C. Wang, L. Li and R. F. Mansour, "Face image compression and reconstruction based on improved pca," Intelligent Automation & Soft Computing, vol. 30, no.3, pp. 973–982, 2021. https://doi.org/10.32604/iasc.2021.017607Citations
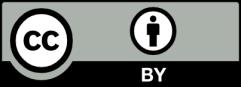