Open Access
ARTICLE
Morphological Feature Aware Multi-CNN Model for Multilingual Text Recognition
1 Shanghai Maritime University, Shanghai, 201306, China
2 University of Pittsburgh, Pittsburgh, PA, 15260, USA
* Corresponding Author: Jin Liu. Email:
Intelligent Automation & Soft Computing 2021, 30(2), 715-733. https://doi.org/10.32604/iasc.2021.020184
Received 13 May 2021; Accepted 14 June 2021; Issue published 11 August 2021
Abstract
Text recognition is a crucial and challenging task, which aims at translating a cropped text instance image into a target string sequence. Recently, Convolutional neural networks (CNN) have been widely used in text recognition tasks as it can effectively capture semantic and structural information in text. However, most existing methods are usually based on contextual clues. If only recognize a single character, the accuracy of these approaches can be reduced. For example, it is difficult to distinguish 0 and O in the traditional CNN network because they are very similar in composition and structure. To solve this problem, we propose a novel neural network model called Morphological Feature Aware Multi-CNN Model for Multilingual Text Recognition (MFAM-CNN) in this article. We introduce a contour extraction model to enrich the representation ability, which can distinguish characters with similar shapes. Self-adaptive text density classification module is designed to recognize characters of different densities, improving the accuracy of character recognition. In general, the model is more sensitive to the overall size of the text, which improves the recognition rate of similar text. To evaluate the effectiveness of our approach, we make a dataset containing Chinese, numbers, and letters called SC dataset. Extensive experiments are conducted on the above SC dataset and ARTI-TEXT dataset, the results demonstrate that our model significantly improves the performance, achieving 98.03% and 97.77% respectively.Keywords
Cite This Article
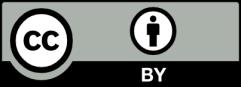
This work is licensed under a Creative Commons Attribution 4.0 International License , which permits unrestricted use, distribution, and reproduction in any medium, provided the original work is properly cited.