Open Access
ARTICLE
Visual Saliency Prediction Using Attention-based Cross-modal Integration Network in RGB-D Images
1 School of Computer Science and Cyberspace Security, Hainan University, 570228, Haikou, China
2 School of Information and Electronic Engineering, Zhejiang University of Science & Technology, 310023, Zhejiang, China
3 Department of Computer Science and Engineering, Michigan State University, 48913, Michigan, USA
* Corresponding Author: Ting Jin. Email:
Intelligent Automation & Soft Computing 2021, 30(2), 439-452. https://doi.org/10.32604/iasc.2021.018643
Received 14 March 2021; Accepted 15 April 2021; Issue published 11 August 2021
Abstract
Saliency prediction has recently gained a large number of attention for the sake of the rapid development of deep neural networks in computer vision tasks. However, there are still dilemmas that need to be addressed. In this paper, we design a visual saliency prediction model using attention-based cross-model integration strategies in RGB-D images. Unlike other symmetric feature extraction networks, we exploit asymmetric networks to effectively extract depth features as the complementary information of RGB information. Then we propose attention modules to integrate cross-modal feature information and emphasize the feature representation of salient regions, meanwhile neglect the surrounding unimportant pixels, so as to reduce the lost of channel details during the feature extraction. Moreover, we contribute successive dilated convolution modules to reduce training parameters and to attain multi-scale reception fields by using dilated convolution layers, also, the successive dilated convolution modules can promote the interaction of two complementary information. Finally, we build the decoder process to explore the continuity and attributes of different levels of enhanced features by gradually concatenating outputs of proposed modules and obtaining final high-quality saliency prediction maps. Experimental results on two widely-agreed datasets demonstrate that our model outperforms than other six state-of-the-art saliency models according to four measure metrics.Keywords
Cite This Article
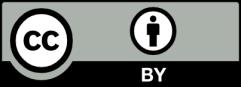
This work is licensed under a Creative Commons Attribution 4.0 International License , which permits unrestricted use, distribution, and reproduction in any medium, provided the original work is properly cited.