Open Access
ARTICLE
Conveyor Belt Detection Based on Deep Convolution GANs
1 College of Information and Computer, Taiyuan University of Technology, Jinzhong, 030600, China
2 State Grid Taiyuan Power Supply Company, Taiyuan, 030012, China
3 International Business Machines Corporation (IBM), New York, NY, USA
* Corresponding Author: Xiaoli Hao. Email:
Intelligent Automation & Soft Computing 2021, 30(2), 601-613. https://doi.org/10.32604/iasc.2021.017963
Received 19 February 2021; Accepted 23 May 2021; Issue published 11 August 2021
Abstract
The belt conveyor is essential in coal mine underground transportation. The belt properties directly affect the safety of the conveyor. It is essential to monitor that the belt works well. Traditional non-contact detection methods are usually time-consuming, and they only identify a single instance of damage. In this paper, a new belt-tear detection method is developed, characterized by two time-scale update rules for a multi-class deep convolution generative adversarial network. To use this method, only a small amount of image data needs to be labeled, and batch normalization in the generator must be removed to avoid artifacts in the generated images. The output of the discriminator uses a multi-classification softmax function to identify the scratches, cracks, and tears in the belt. In addition, we have improved the two time-scale update rule, by which the generator and discriminator use different learning rates, updated it according to , and defined as 2:1. It can strike a balance between the generator and discriminator, speed up discriminator training, and improve real-time damage detection. Experimental results show that the detection accuracy of tears reaches 100%, and the detection accuracy of non-serious damage is up to 97.1%.Keywords
Cite This Article
X. Hao, X. Meng, Y. Zhang, J. Xue and J. Xia, "Conveyor belt detection based on deep convolution gans," Intelligent Automation & Soft Computing, vol. 30, no.2, pp. 601–613, 2021. https://doi.org/10.32604/iasc.2021.017963Citations
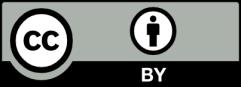