Open Access
ARTICLE
An Adversarial Network-based Multi-model Black-box Attack
1 Sichuan Normal University, Chengdu, 610066, China
2 School of Computer Science, Southwest Petroleum University, Chengdu, 610500, China
3 AECC Sichuan Gas Turbine Establishment, Mianyang, 621700, China
4 Brunel University London, Uxbridge, Middlesex, UB83PH, United Kingdom
5 Institute of Logistics Science and Technology, Beijing, 100166, China
* Corresponding Author: Wangchi Cheng. Email:
Intelligent Automation & Soft Computing 2021, 30(2), 641-649. https://doi.org/10.32604/iasc.2021.016818
Received 12 January 2021; Accepted 27 April 2021; Issue published 11 August 2021
Abstract
Researches have shown that Deep neural networks (DNNs) are vulnerable to adversarial examples. In this paper, we propose a generative model to explore how to produce adversarial examples that can deceive multiple deep learning models simultaneously. Unlike most of popular adversarial attack algorithms, the one proposed in this paper is based on the Generative Adversarial Networks (GAN). It can quickly produce adversarial examples and perform black-box attacks on multi-model. To enhance the transferability of the samples generated by our approach, we use multiple neural networks in the training process. Experimental results on MNIST showed that our method can efficiently generate adversarial examples. Moreover, it can successfully attack various classes of deep neural networks at the same time, such as fully connected neural networks (FCNN), convolutional neural networks (CNN) and recurrent neural networks (RNN). We performed a black-box attack on VGG16 and the experimental results showed that when the test data classes are ten (0–9), the attack success rate is 97.68%, and when the test data classes are seven (0–6), the attack success rate is up to 98.25%.Keywords
Cite This Article
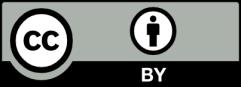
This work is licensed under a Creative Commons Attribution 4.0 International License , which permits unrestricted use, distribution, and reproduction in any medium, provided the original work is properly cited.