Open Access
ARTICLE
Robust Sound Source Localization Using Convolutional Neural Network Based on Microphone Array
1 School of Information and Communication Engineering, Nanjing Institute of Technology, Nanjing, 211167, China
2 School of Information Science and Engineering, Southeast University, Nanjing, 210096, China
3 University of Oulu, Oulu, 900014, FI, Finland
* Corresponding Author: Xiaoyan Zhao. Email:
Intelligent Automation & Soft Computing 2021, 30(1), 361-371. https://doi.org/10.32604/iasc.2021.018823
Received 22 March 2021; Accepted 23 April 2021; Issue published 26 July 2021
Abstract
In order to improve the performance of microphone array-based sound source localization (SSL), a robust SSL algorithm using convolutional neural network (CNN) is proposed in this paper. The Gammatone sub-band steered response power-phase transform (SRP-PHAT) spatial spectrum is adopted as the localization cue due to its feature correlation of consecutive sub-bands. Since CNN has the “weight sharing” characteristics and the advantage of processing tensor data, it is adopted to extract spatial location information from the localization cues. The Gammatone sub-band SRP-PHAT spatial spectrum are calculated through the microphone signals decomposed in frequency domain by Gammatone filters bank. The proposed algorithm takes a two-dimensional feature matrix which is assembled from Gammatone sub-band SRP-PHAT spatial spectrum within a frame as CNN input. Taking the advantage of powerful modeling capability of CNN, the two-dimensional feature matrices in diverse environments are used together to train the CNN model which reflects mapping regularity between the feature matrix and the azimuth of sound source. The estimated azimuth of the testing signal is predicted through the trained CNN model. Experimental results show the superiority of the proposed algorithm in SSL problem, it achieves significantly improved localization performance and capacity of robustness and generality in various acoustic environments.Keywords
Cite This Article
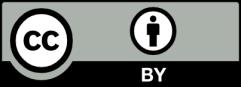
This work is licensed under a Creative Commons Attribution 4.0 International License , which permits unrestricted use, distribution, and reproduction in any medium, provided the original work is properly cited.