Open Access
ARTICLE
A Shadowed Rough-fuzzy Clustering Algorithm Based on Mahalanobis Distance for Intrusion Detection
1 School of Artificial Intelligence, Nanjing University of Information Science and Technology, Nanjing, 210044, China
2 Southern Marine Science and Engineering Guangdong Laboratory (Zhuhai), Zhuhai, 519080, China
3 School of Electronic and Information Engineering, Nanjing University of Information Science and Technology, Nanjing, 210044, China
4 School of Computer and software, Nanjing University of Information Science and Technology, Nanjing, 210044, China
5 International Business Machines Corporation (IBM), New York, NY, 10504, USA
* Corresponding Author: Lina Wang. Email:
Intelligent Automation & Soft Computing 2021, 30(1), 31-47. https://doi.org/10.32604/iasc.2021.018577
Received 12 March 2021; Accepted 23 April 2021; Issue published 26 July 2021
Abstract
Intrusion detection has been widely used in many application domains; thus, it has caught significant attention in academic fields these years. Assembled with more and more sub-systems, the network is more vulnerable to multiple attacks aiming at the network security. Compared with the other issues such as complex environment and resources-constrained devices, network security has been the biggest challenge for Internet construction. To deal with this problem, a fundamental measure for safeguarding network security is to select an intrusion detection algorithm. As is known, it is less effective to determine the abnormal behavior as an intrusion and learn the entire scope of the normal behavior with the traditional anomaly-based algorithm for Internet intrusion detection. In this paper, we propose an intrusion-detecting algorithm of shadowed rough-fuzzy clustering based on Mahalanobis distance, named MSRFCM. It adopts dissimilarity measurement of Mahalanobis distance to identify the relevant variables that significantly influence the clustering performance and reduce the error rate in the process of partitioning clusters with high attribute correlation. And shadowed rough-fuzzy clustering (SRFCM) is applied to obtaining real value-approaching prototypes based on iteration and partitioning the data set into more meaningful clusters. Through simulation with the NSL-KDD intrusion data set and three other intrusion data sets, the Mahalanobis distance-based shadowed rough-fuzzy clustering algorithm has improved performance in intrusion detection.Keywords
Cite This Article
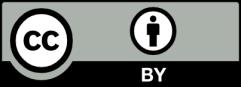