Open Access
ARTICLE
A Hybrid Deep Learning Intrusion Detection Model for Fog Computing Environment
Department of Computer Science and Engineering, E.G.S. Pillay Engineering College, Nagapattinam, 611002, India
* Corresponding Author: K. Kalaivani. Email:
Intelligent Automation & Soft Computing 2021, 30(1), 1-15. https://doi.org/10.32604/iasc.2021.017515
Received 01 February 2021; Accepted 16 April 2021; Issue published 26 July 2021
Abstract
Fog computing extends the concept of cloud computing by providing the services of computing, storage, and networking connectivity at the edge between data centers in cloud computing environments and end devices. Having the intelligence at the edge enables faster real-time decision-making and reduces the amount of data forwarded to the cloud. When enhanced by fog computing, the Internet of Things (IoT) brings low latency and improves real time and quality of service (QoS) in IoT applications of augmented reality, smart grids, smart vehicles, and healthcare. However, both cloud and fog computing environments are vulnerable to several kinds of attacks that can lead to unexpected loss. For example, a denial of service (DoS) attack can block authenticated users by rendering network resources unavailable and consuming network bandwidth unnecessarily. This paper proposes an intrusion classification model using a convolutional neural network (CNN) and Long Short-Term Memory networks (LSTM) to obtain the advantages of deep learning methods in order to accurately predict such attacks. The proposed integrated CNN with LSTM-based Fog Computing Intrusion Detection ICNN-FCID model is used for multi-class attack classification. Our proposed model is demonstrated using NSL-KDD, a benchmark dataset, and provides attack detection accuracy of about 96.5%. Comparisons of the accuracy of our model with both traditional and other recent deep learning approaches show that our model is superior in performance. The ICNN-FCID model can be used in fog layer devices where network traffic is monitored and the attacks are detected. As a result, the cloud server and fog layer devices can be protected from malicious users and are always available in providing services to IoT devices.Keywords
Cite This Article
Citations
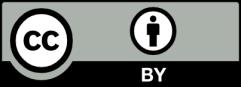
This work is licensed under a Creative Commons Attribution 4.0 International License , which permits unrestricted use, distribution, and reproduction in any medium, provided the original work is properly cited.