Open Access
ARTICLE
Exploiting Rich Event Representation to Improve Event Causality Recognition
1 School of Computer and Electronic Information, Nanjing Normal University, Nanjing, 210046, China
2 School of Computer Science and Electronic Engineering, University of Essex, Colchester, UK
* Corresponding Author: Junsheng Zhou. Email:
Intelligent Automation & Soft Computing 2021, 30(1), 161-173. https://doi.org/10.32604/iasc.2021.017440
Received 30 January 2021; Accepted 16 March 2021; Issue published 26 July 2021
Abstract
Event causality identification is an essential task for information extraction that has attracted growing attention. Early researchers were accustomed to combining the convolutional neural network or recurrent neural network models with external causal knowledge, but these methods ignore the importance of rich semantic representation of the event. The event is more structured, so it has more abundant semantic representation. We argue that the elements of the event, the interaction of the two events, and the context between the two events can enrich the event’s semantic representation and help identify event causality. Therefore, the effective semantic representation of events in event causality recognition deserves further study. To verify the effectiveness of rich event semantic representation for event causality identification, we proposed a model exploiting rich event representation to improve event causality recognition. Our model is based on multi-column convolutional neural networks, which integrate rich event representation, including event tensor representation, event interaction representation, and context-aware event representation. We designed various experimental models and conducted experiments on the Chinese emergency corpus, the most comprehensive annotation of events and event elements, enabling us to study the semantic representation of events from all aspects. The extensive experiments showed that the rich semantic representation of events achieved significant performance improvement over the baseline model on event causality recognition, indicating that the semantic representation of events plays an important role in event causality recognition.Keywords
Cite This Article
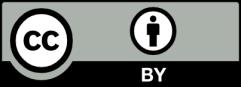