Open Access
ARTICLE
Detection of COVID-19 Using Deep Learning on X-Ray Images
1 Department of Computer Science, Shaqra University, Shaqra, Saudi Arabia
2 Department of Information Technology, and Sensor Networks and Cellular Systems Research Center (SNCS), University of Tabuk, Tabuk, Saudi Arabia
* Corresponding Author: Munif Alotaibi. Email:
Intelligent Automation & Soft Computing 2021, 29(3), 885-898. https://doi.org/10.32604/iasc.2021.018350
Received 05 March 2021; Accepted 22 April 2021; Issue published 01 July 2021
Abstract
The novel coronavirus 2019 (COVID-19) is one of a large family of viruses that cause illness, the symptoms of which range from a common cold, fever, coughing, and shortness of breath to more severe symptoms. The virus rapidly and easily spreads from infected people to others through close contact in the absence of protection. Early detection of COVID-19 assists governmental authorities and healthcare specialists in reducing the chain of transmission and flattening the curve of the pandemic. The widespread form of the COVID-19 diagnostic test lacks a high true positive rate and a low false negative rate and needs a particular piece of hardware. Multifariously, computed tomography (CT) and X-ray images indicate evidence of COVID-19 disease. However, it is difficult to diagnose COVID-19 owing to the overlap of its symptoms with those of other lung infections. Thus, there is an urgent need for precise diagnostic solutions based on deep learning algorithms, such as convolutional neural networks, to quickly detect positive COVID-19 cases. Therefore, we propose a deep learning method based on an advanced convolutional neural network architecture known as EffencientNet pre-trained on ImageNet dataset to detect COVID-19 from chest X-ray images. The proposed method improves the accuracy of existing state-of-the-art techniques and can be used by medical staff to screen patients. The framework is trained and validated using 1,125 chest raw X-ray images (i.e., these images are not in regular shape and require image preprocessing) and compared with existing techniques. Three sets of experiments were carried out to detect COVID-19 early utilizing raw X-ray images. For the three-class (i.e., COVID-19, pneumonia, and no-findings) classification set of experiments, our method achieved an average accuracy of 89.60%. For the binary classification two set of experiments, our method yielded an average accuracy of 99.04% and 99.11%. The proposed method has achieved superior results compared to state-of-art methods. The method is a candidate solution for accurately detecting positive COVID-19 cases as soon as they occur using X-ray images. Additionally, the method does not require extensive preprocessing or feature extraction. The proposed technique can be utilized in remote areas where radiologists are not available and can detect other chest-related diseases, such as pneumonia.Keywords
Cite This Article
Citations
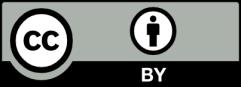
This work is licensed under a Creative Commons Attribution 4.0 International License , which permits unrestricted use, distribution, and reproduction in any medium, provided the original work is properly cited.