Open Access
ARTICLE
Forecast of LSTM-XGBoost in Stock Price Based on Bayesian Optimization
1 Macau University of Science and Technology, Taipa, 999078, Macau
2 Guangdong University of Science and Technology, Dongguan, 523083, China
3 Guangdong University of Technology, Guangzhou, 510006, China
4 Ankang Vocational Technical College, Ankang, 725000, China
* Corresponding Author: Tian Liwei. Email:
Intelligent Automation & Soft Computing 2021, 29(3), 855-868. https://doi.org/10.32604/iasc.2021.016805
Received 12 January 2021; Accepted 14 April 2021; Issue published 01 July 2021
Abstract
The prediction of the “ups and downs” of stock market prices is one of the important undertakings of the financial market. Since accurate prediction helps foster considerable economic benefits, stock market prediction has attracted significant interest by both investors and researchers. Efforts into building an accurate, stable and effective model to predict stock prices’ movements have been proliferating at a fast pace, to meet such a challenge. Firstly, this paper uses a correlation analysis to analyze the attributes of a stock dataset, processing missing values, determining the data attributes to be retained data, then divide it in a training set and a testing set. Then, the LSTM model is subsequently used to predict the retained attributes after the analysis and retention of prediction results to construct a new testing set. At the same time, the Bo-XGBoost model based on XGBoost is used to train the original training set. It uses Bayesian calculation to optimize the parameters which are proved to be difficult to find the best solution in the XGBoost model. Finally, the LSTM-BO-XGBoost model proposed in this paper is applied to “ES=F”, “YM=F”, “CL=F”, “^TNX”, “^N225”, “NQ=F”, “AAPL”, “GC= F”, “JPY=X” and “SI=F” rates with 10 stocks in the forecast and evaluated by four evaluation indexes: root mean square error (RMSE), average absolute error (MAE), accuracy rate, and F1-score. It is found that the LSTM-BO-XGBoost model proposed in this paper performs better than LSTM in stock price prediction. In order to further evaluate the performance of the algorithm, the LSTM-BO-XGBoost mode is compared with the single LSTM network model and RNN network model, the LSTM-BO-XGBoost hybrid model. The results show that the LSTM-BO-XGBoost mode has high performance, stability and feasibility than the others.Keywords
Cite This Article
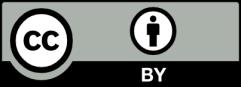