Open Access
ARTICLE
Design and Experimentation of Causal Relationship Discovery among Features of Healthcare Datasets
Department of CSE, Pondicherry Engineering College, Puducherry, 605 014, India
* Corresponding Author: Y. Sreeraman. Email:
Intelligent Automation & Soft Computing 2021, 29(2), 539-557. https://doi.org/10.32604/iasc.2021.017256
Received 25 January 2021; Accepted 03 April 2021; Issue published 16 June 2021
Abstract
Causal relationships in a data play vital role in decision making. Identification of causal association in data is one of the important areas of research in data analytics. Simple correlations between data variables reveal the degree of linear relationship. Partial correlation explains the association between two variables within the control of other related variables. Partial association test explains the causality in data. In this paper a couple of causal relationship discovery strategies are proposed using the design of partial association tree that makes use of partial association test among variables. These decision trees are different from normal decision trees in terms of construction, scalability, interpretability and the ability of identifying causality in data. Normal Decision Trees are supervised machine learning approach to classify data based on a labeled attribute values. Variants of partial association trees are constructed as a part of analytics on a number of healthcare datasets. The applicability of design variants are carefully analyzed through this experimentation. In the above said experimentation it is found that the causation in data is not existed in data in some situations and sometimes the existing causality cannot be extracted where low associated dimensions are involved in data and hiding the underlying causality. One of the variants of proposed algorithms which was named dimensionality reduced partial association tree, did well in extracting causal association in case of a hidden causality in data.Keywords
Cite This Article
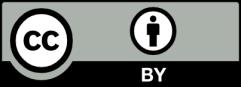
This work is licensed under a Creative Commons Attribution 4.0 International License , which permits unrestricted use, distribution, and reproduction in any medium, provided the original work is properly cited.