Open Access
ARTICLE
Handwritten Character Recognition Based on Improved Convolutional Neural Network
1 School of Computer and Software, Nanjing University of Information Science and Technology, Nanjing, 210044, China
2 Jiangsu Key Laboratory of Data Science and Smart Software, Jinling Institute of Technology, Nanjing, 211169, China
3 Department of Electronics and Computer Science Koszalin University of Technology Sniadeckich 2, 75-453, Koszalin, Poland
4 Faculty of Business, Australian College of Kuwait, State of Kuwait
* Corresponding Author: Yu Xue. Email:
Intelligent Automation & Soft Computing 2021, 29(2), 497-509. https://doi.org/10.32604/iasc.2021.016884
Received 14 January 2021; Accepted 23 March 2021; Issue published 16 June 2021
Abstract
Because of the characteristics of high redundancy, high parallelism and nonlinearity in the handwritten character recognition model, the convolutional neural networks (CNNs) are becoming the first choice to solve these complex problems. The complexity, the types of characters, the character similarity of the handwritten character dataset, and the choice of optimizers all have a great impact on the network model, resulting in low accuracy, high loss, and other problems. In view of the existence of these problems, an improved LeNet-5 model is proposed. Through increasing its convolutional layers and fully connected layers, higher quality features can be extracted. Secondly, a more complex dataset called EMNIST is selected and many experiments are carried out. After many experiments, the Adam optimization algorithm is finally chosen to optimize the network model. Then, for processing character similarity problems on the pre-processed EMNIST dataset, the dataset is divided into different parts and to be processed. A better-divided result is selected after the comparative experiments. Finally, the high accuracy recognition of handwritten characters is achieved. The experimental results show that the recognition accuracy of the handwritten characters reached at 88% in the test set, and the loss is low.Keywords
Cite This Article
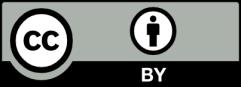
This work is licensed under a Creative Commons Attribution 4.0 International License , which permits unrestricted use, distribution, and reproduction in any medium, provided the original work is properly cited.