Open Access
ARTICLE
Evaluating and Ranking Mobile Learning Factors Using a Multi-criterion Decision-making (MCDM) Approach
1 College of Computer Science, King Khalid University, Abha, 61413, Saudi Arabia
2 Department of Computer Science, Bahria University Lahore Campus, Punjab, 54600, Pakistan
3 College of Engineering, King Khalid University, Abha, 61413, Saudi Arabia
4 Department of Software Engineering, University of Gujrat, Punjab, 50700, Pakistan
5 College of Science & Arts Rejal Almah, King Khalid University, Abha, 62529, Saudi Arabia
* Corresponding Author: Ansar Siddique. Email:
(This article belongs to the Special Issue: Soft Computing Methods for Innovative Software Practices)
Intelligent Automation & Soft Computing 2021, 29(1), 111-129. https://doi.org/10.32604/iasc.2021.015009
Received 02 November 2020; Accepted 28 February 2021; Issue published 12 May 2021
Abstract
The escalating growth in digital technology is setting the stage for changes in university education, as E-learning brings students and faculties outside the contained classroom environment. While mobile learning is considered an emerging technology, there is comprehensive literature on mobile learning and its applications. However, there has been relatively little research on mobile learning recognition and readiness compared to mobile learning studies and implementations. The advent of mobile learning (M-learning) provides additional flexibility in terms of time and location. M-learning lacks an established place in university education. The influence of its critical success factors (CSFs) on the university education system must be analyzed and understood. In the present study, decision-makers establish four dimensions which are further classified into 13 CSFs to evaluate and rank them. It is imperative to judge the most important CSFs and rank them according to their importance. To this end, multi-criteria decision-making (MCDM), like the fuzzy analytic hierarchy process (FAHP), is an important tool to establish the influence of each CSF. It identifies the four dimensions of M-learning by evaluation in a crisp and fuzzy environment. Global and local weights have been employed for ranking in a decision-making process to enable universities to choose the best adoption factor for mobile learning. The result establishes the influence of CSFs in M-learning success, in decreasing order, as the technological dimension (TD), individual/user dimension (ID), pedagogical dimension (PD), and social dimension (SD). A greater understanding of the mobile learning implementation process can allow researchers and decision-makers to collaborate to incorporate effective mobile learning strategies.Keywords
Cite This Article
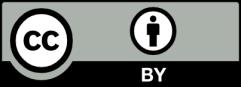
This work is licensed under a Creative Commons Attribution 4.0 International License , which permits unrestricted use, distribution, and reproduction in any medium, provided the original work is properly cited.