Open Access
ARTICLE
A Rock-fall Early Warning System Based on Logistic Regression Model
1 Department of Computer Science, College of Science, King Khalid University, Muhayil, 63772, Saudi Arabia
2 Department of Information System, College of Science, King Khalid University, Muhayil, 63772, Saudi Arabia
3 Faculty of Engineering, Department of Electrical Engineering, Dongola University, Dongola, 41129, Sudan
4 Department of Management Information System, College of Business, King Khalid University, Abba, 61421, Saudi Arabia
* Corresponding Author: Mohammed Abaker. Email:
Intelligent Automation & Soft Computing 2021, 28(3), 843-856. https://doi.org/10.32604/iasc.2021.017714
Received 05 February 2021; Accepted 08 March 2021; Issue published 20 April 2021
Abstract
The rock-fall is a natural hazard that results in many economic damages and human losses annually, and thus proactive policies to prevent rock-fall hazard are needed. Such policies require predicting the rock-fall occurrence and deciding to alert the road users at the appropriate time. Thus, this study develops a rock-fall early warning system based on logistic regression model. In this system, the logistic regression model is used to predict the rock-fall occurrence. The decision-making algorithm is used to classify the hazard levels and delivers early warning action. This study adopts two criteria to evaluate the system predictive performance, including overall prediction accuracy measures based on a confusion matrix and the area under a receiver operating characteristic curve (AUC). The results show that the correct prediction accuracy was approximately 79.9%, and the area under the curve (AUC) was 0.85 during the model training. During the validation process, the overall accuracy is 81.0%, and (AUC) is 0.90. The result indicates that this system has high predictive power, strong robustness, and stable performance. That confirms the usefulness of a logistic regression model for predicting a rock-fall occurrence probability.Keywords
Cite This Article
Citations
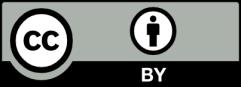