Open Access
ARTICLE
CAMNet: DeepGait Feature Extraction via Maximum Activated Channel Localization
Computer Engineering Department, Cyprus International University, Nicosia, North Cyprus, Mersin 10, 099010, Turkey
* Corresponding Author: Salisu Muhammed. Email:
Intelligent Automation & Soft Computing 2021, 28(2), 397-416. https://doi.org/10.32604/iasc.2021.016574
Received 05 January 2021; Accepted 05 February 2021; Issue published 01 April 2021
Abstract
As the models with fewer operations help realize the performance of intelligent computing systems, we propose a novel deep network for DeepGait feature extraction with less operation for video sensor-based gait representation without dimension decomposition. The DeepGait has been known to have outperformed the hand-crafted representations, such as the frequency-domain feature (FDF), gait energy image (GEI), and gait flow image (GFI), etc. More explicitly, the channel-activated mapping network (CAMNet) is composed of three progressive triplets of convolution, batch normalization, max-pooling layers, and an external max pooling to capture the Spatio-temporal information of multiple frames in one gait period. We conducted experiments to validate the effectiveness of the proposed novel algorithm in terms of cross-view gait recognition in both cooperative and uncooperative settings using the state-of-the-art OU-ISIR multi-view large population OU-MVLP dataset. The OU-MVLP dataset includes 10307 subjects. As a result, we confirmed that the proposed method significantly outperformed state-of-the-art approaches using the same dataset at the rear angles of 180, 195, 210, and 225, in both cooperative and uncooperative settings for verification scenarios.Keywords
Cite This Article
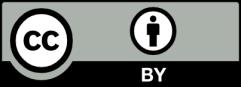