Open Access
ARTICLE
Oral English Speech Recognition Based on Enhanced Temporal Convolutional Network
1 Hunan Radio and TV University, Changsha, 410004, China
2 School of Computing Science and Engineering, Vellore Institute of Technology, Tamil Nadu, 632014, India
* Corresponding Author: Hao Wu. Email:
Intelligent Automation & Soft Computing 2021, 28(1), 121-132. https://doi.org/10.32604/iasc.2021.016457
Received 02 January 2021; Accepted 02 February 2021; Issue published 17 March 2021
Abstract
In oral English teaching in China, teachers usually improve students’ pronunciation by their subjective judgment. Even to the same student, the teacher gives different suggestions at different times. Students’ oral pronunciation features can be obtained from the reconstructed acoustic and natural language features of speech audio, but the task is complicated due to the embedding of multimodal sentences. To solve this problem, this paper proposes an English speech recognition based on enhanced temporal convolution network. Firstly, a suitable UNet network model is designed to extract the noise of speech signal and achieve the purpose of speech enhancement. Secondly, a network model with stable parameters is obtained by pre training, which is helpful to distinguish the spoken speech signals. Thirdly, a temporal convolution network with residual connection is designed to infer the meaning of pronunciation. Finally, the speech is graded according to the difference between the output value and the real result, according to the details of students’ oral pronunciation, the intelligent guidance of students’ oral pronunciation can be realized. The experimental results show that the model file obtained after training is improved under the controlling of file size. From the test results of LibriSpeech ASR corpus, it demonstrates the effectiveness and advantage of this approach.Keywords
Cite This Article
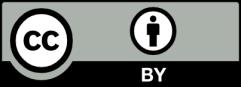