Open Access
ARTICLE
Hybrid Deep Learning Modeling for Water Level Prediction in Yangtze River
1 School of Energy and Power Engineering, Wuhan University of Technology, Wuhan, 430070, China
2 School of Automation, Wuhan University of Technology, Wuhan, 430070, China
3 University of New South Wales, Sydney, NSW, 2052, Australia
* Corresponding Author: Zhaoqing Xie. Email:
Intelligent Automation & Soft Computing 2021, 28(1), 153-166. https://doi.org/10.32604/iasc.2021.016246
Received 28 December 2020; Accepted 06 February 2021; Issue published 17 March 2021
Abstract
Accurate prediction of water level in inland waterway has been an important issue for helping flood control and vessel navigation in a proactive manner. In this research, a deep learning approach called long short-term memory network combined with discrete wavelet transform (WA-LSTM) is proposed for daily water level prediction. The wavelet transform is applied to decompose time series into details and approximation components for a better understanding of temporal properties, and a novel LSTM network is used to learn generic water level features through layer-by-layer feature granulation with a greedy layer wise unsupervised learning algorithm. Six representative reaches in Yangtze River, namely, the Jianli, Wuhan, Jiujiang, Anqing, Wuhu, and Nanjing are investigated, and water level data from 2010 to 2019 are processed through temporal and spatial correlation analysis, and combination-optimized to develop and evaluate the proposed model. In general, the test average performances on RMSE and MAE are less than 0.045 m and 0.035 m respectively, which outperforms the state-of-the-art models, such as WA-ANN, WA-ARIMA and LSTM models. The results indicate that the WA-LSTM model is stable, reliable and widely applicable.Keywords
Cite This Article
Citations
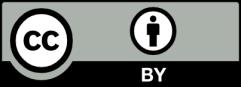
This work is licensed under a Creative Commons Attribution 4.0 International License , which permits unrestricted use, distribution, and reproduction in any medium, provided the original work is properly cited.