Open Access
ARTICLE
Filter-Based Feature Selection and Machine-Learning Classification of Cancer Data
College of Computer Science and Engineering, Taibah University, Yanbu, Saudi Arabia
* Corresponding Author: Mohammed Farsi. Email:
Intelligent Automation & Soft Computing 2021, 28(1), 83-92. https://doi.org/10.32604/iasc.2021.015460
Received 22 November 2020; Accepted 02 February 2021; Issue published 17 March 2021
Abstract
Microarray cancer data poses many challenges for machine-learning (ML) classification including noisy data, small sample size, high dimensionality, and imbalanced class labels. In this paper, we propose a framework to address these problems by properly utilizing feature-selection techniques. The most important features of the cancer datasets were extracted with Logistic Regression (LR), Chi-2, Random Forest (RF), and LightGBM. These extracted features served as input columns in an applied classification task. This framework’s main advantages are reducing time complexity and the number of irrelevant features for the dataset. For evaluation, the proposed method was compared to models using Support Vector Machine (SVM), k-Nearest Neighbor (KNN), Decision Tree (DT), LR, and RF. To prove the proposed framework’s efficiency, all the experiments were performed on four standard datasets, encompassing two binary and two multiclass imbalanced-microarray cancer datasets: Lung (5-class dataset), Small Round Blue Cell Tumors (SRBCT; 4-class dataset), and Ovarian and Breast Cancer 2-class datasets). The experimental results of our comparison showed that the proposed framework achieved the highest predictive performance. A comparative study of our framework, using accuracy and F1 as metrics, was performed against state-of-the-art approacheswhich illustrated that the proposed method presented a better result for two of the selected datasets.Keywords
Cite This Article
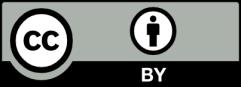
This work is licensed under a Creative Commons Attribution 4.0 International License , which permits unrestricted use, distribution, and reproduction in any medium, provided the original work is properly cited.