Open Access
ARTICLE
Automatic BIM Indoor Modelling from Unstructured Point Clouds Using a Convolutional Neural Network
Department of Computer Science and Engineering, Seoul National University of Science and Technology, Seoul, Korea
* Corresponding Author: Ji-Hyeong Han. Email:
Intelligent Automation & Soft Computing 2021, 28(1), 133-152. https://doi.org/10.32604/iasc.2021.015227
Received 11 November 2020; Accepted 03 February 2021; Issue published 17 March 2021
Abstract
The automated reconstruction of building information modeling (BIM) objects from unstructured point cloud data for indoor as-built modeling is still a challenging task and the subject of much ongoing research. The most important part of the process is to detect the wall geometry clearly. A popular method is first to segment and classify point clouds, after which the identified segments should be clustered according to their corresponding objects, such as walls and clutter. To perform this process, a major problem is low-quality point clouds that are noisy, cluttered and that contain missing parts in the data. Moreover, the size of the data introduces significant computational challenges. In this paper, we propose a fully automated and efficient method to reconstruct as-built BIM objects. First, we propose an input point cloud preprocessing method for reconstruction accuracy and efficiency. It consists of a simplification step and an upsampling step. In the simplification step, the input point clouds are simplified to denoise and remove certain outliers without changing the innate structure or orientation information. In the upsampling step, the new points are generated for the simplified point clouds to fill missing parts in the plane and nearby edges. Second, a 2D depth image is generated based on the preprocessed point clouds after which we apply a convolutional neural network (CNN) to detect the wall topology. Moreover, we detect doors in each detected wall using a proposed template matching algorithm. Finally, the BIM object is reconstructed with the detected walls and doors geometry by creating IfcWallStrandardCase and IfcDoor objects in the IFC4 standard. Experiments based on residual house point cloud datasets prove that the proposed method is reliable for wall and door reconstruction from unstructured point clouds. As a result, with the detected walls and doors, 95% of the data is successfully identified.Keywords
Cite This Article
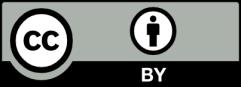