Open Access
ARTICLE
Weed Recognition for Depthwise Separable Network Based on Transfer Learning
1 College of Information and Technology, Jilin Agricultural University, Changchun, 130118, China
2 Changchun Institute of Engineering and Technology, Changchun, 130117, China
3 Department of Biological Systems Engineering, Centre for Precision and Automated Agricultural Systems, Washington State University, Prossers, WA, 99350, USA
* Corresponding Author: Yang Zhou. Email:
Intelligent Automation & Soft Computing 2021, 27(3), 669-682. https://doi.org/10.32604/iasc.2021.015225
Received 11 November 2020; Accepted 22 December 2020; Issue published 01 March 2021
Abstract
For improving the accuracy of weed recognition under complex field conditions, a weed recognition method using depthwise separable convolutional neural network based on deep transfer learning was proposed in this study. To improve the model classification accuracy, the Xception model was refined by using model transferring and fine-tuning. Specifically, the weight parameters trained by ImageNet data set were transferred to the Xception model. Then a global average pooling layer replaced the full connection layer of the Xception model. Finally, the XGBoost classifier was added to the top layer of the model to output results. The performance of the proposed model was validated using the digital field weed images. The experimental results demonstrated that the proposed method had significant improvement in both classification accuracy and training speed in comparison of VGG16, ResNet50, and Xception depth models. The test recognition accuracy of the proposed model reached to 99.63%. Further, the training of each round time cost was 208 s, less than VGG16, ResNet50 and Xception models’, which were 248 s, 245 s and 217 s, respectively. Therefore, the proposed model has a promising ability to process image detection and output more accurate recognition results, which can be used for other crops’ precision management.Keywords
Cite This Article
Citations
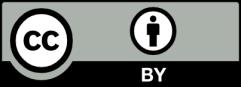
This work is licensed under a Creative Commons Attribution 4.0 International License , which permits unrestricted use, distribution, and reproduction in any medium, provided the original work is properly cited.