Open Access
ARTICLE
Mammographic Image Classification Using Deep Neural Network for Computer-Aided Diagnosis
1 Department of Electronics and Communication Engineering, Government College of Engineering, Bargur, Tamil Nadu, India
2 Department of Computer Science and Engineering, SRM Institute of Science and Technology, Kattankulathur, 603203, Tamil Nadu, India
3 Data Scientist at ConverSight.ai, Indiana, USA
4 Department of Computer Science and Engineering, Kalaignar Karunanidhi Institute of Technology, Coimbatore, Tamil Nadu, India
* Corresponding Author: Charles Arputham. Email:
Intelligent Automation & Soft Computing 2021, 27(3), 747-759. https://doi.org/10.32604/iasc.2021.012077
Received 30 August 2020; Accepted 15 November 2020; Issue published 01 March 2021
Abstract
Breast cancer detection is a crucial topic in the healthcare sector. Breast cancer is a major reason for the increased mortality rate in recent years among women, specifically in developed and underdeveloped countries around the world. The incidence rate is less in India than in developed countries, but awareness must be increased. This paper focuses on an efficient deep learning-based diagnosis and classification technique to detect breast cancer from mammograms. The model includes preprocessing, segmentation, feature extraction, and classification. At the initial level, Laplacian filtering is applied to identify the portions of edges in mammogram images that are highly sensitive to noise. Subsequently, segmentation is done using modified adaptively regularized kernel-based fuzzy C means (ARKFCM). Feature extraction is accomplished using the morphological, texture, and moment invariants. The corresponding feature values are provided as inputs to a deep neural network (DNN) model that classifies the normal and abnormal portions in the mammogram images. The performance of the proposed model is validated with the Mammographic Image Analysis Society (MIAS) database. The efficiency of the proposed classifier is experimentally proved by comparing the various classifiers with respect to their statistical performances. On an applied database, the proposed model offered a maximum classification with the highest accuracy level of 99.13%.Keywords
Cite This Article
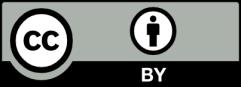
This work is licensed under a Creative Commons Attribution 4.0 International License , which permits unrestricted use, distribution, and reproduction in any medium, provided the original work is properly cited.