Open Access
ARTICLE
Roughsets-based Approach for Predicting Battery Life in IoT
1 Vellore Institute of Technology, Vellore, Tamil Nadu, 632014, India
2 Faculty of Science and Technology, University of the Faroe Islands, Vestarabryggja 15, FO 100, Torshavn, Faroe Islands
* Corresponding Author: Qin Xin. Email:
(This article belongs to the Special Issue: Soft Computing Methods for Innovative Software Practices)
Intelligent Automation & Soft Computing 2021, 27(2), 453-469. https://doi.org/10.32604/iasc.2021.014369
Received 16 September 2020; Accepted 10 November 2020; Issue published 18 January 2021
Abstract
Internet of Things (IoT) and related applications have successfully contributed towards enhancing the value of life in this planet. The advanced wireless sensor networks and its revolutionary computational capabilities have enabled various IoT applications become the next frontier, touching almost all domains of life. With this enormous progress, energy optimization has also become a primary concern with the need to attend to green technologies. The present study focuses on the predictions pertinent to the sustainability of battery life in IoT frameworks in the marine environment. The data used is a publicly available dataset collected from the Chicago district beach water. Firstly, the missing values in the data are replaced with the attribute mean. Later, one-hot encoding technique is applied for achieving data homogeneity followed by the standard scalar technique to normalize the data. Then, rough set theory is used for feature extraction, and the resultant data is fed into a Deep Neural Network (DNN) model for the optimized prediction results. The proposed model is then compared with the state of the art machine learning models and the results justify its superiority on the basis of performance metrics such as Mean Squared Error, Mean Absolute Error, Root Mean Squared Error, and Test Variance Score.Keywords
Cite This Article
Citations
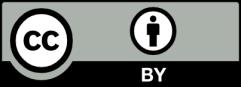