Open Access
ARTICLE
Generation of Synthetic Images of Randomly Stacked Object Scenes for Network Training Applications
1 East China University of Science and Technology, Shanghai, 200237, China
2 Shanghai Aerospace Control Technology Institute, Shanghai, 201109, China
* Corresponding Author: Yajun Zhang. Email:
Intelligent Automation & Soft Computing 2021, 27(2), 425-439. https://doi.org/10.32604/iasc.2021.013795
Received 30 August 2020; Accepted 15 November 2020; Issue published 18 January 2021
Abstract
Image recognition algorithms based on deep learning have been widely developed in recent years owing to their capability of automatically capturing recognition features from image datasets and constantly improving the accuracy and efficiency of the image recognition process. However, the task of training deep learning networks is time-consuming and expensive because large training datasets are generally required, and extensive manpower is needed to annotate each of the images in the training dataset to support the supervised learning process. This task is particularly arduous when the image scenes involve randomly stacked objects. The present work addresses this issue by developing a synthetic training dataset generation method based on OpenGL and the Bullet physics engine which can automatically generate annotated synthetic datasets by simulating the freefall of a collection of objects under the force of gravity. Rigorous statistical comparison of a real image dataset of staked scenes with a synthetic image dataset generated by the proposed approach demonstrates that the two datasets exhibit no significant differences. Moreover, the object detection performances obtained by three popular network architectures trained using the synthetic dataset generated by the proposed approach are demonstrated to be much better than the results of training conducted using a synthetic dataset generated by a conventional cut and paste approach, and these performances are also competitive with the results of training conducted using a dataset composed of real images.Keywords
Cite This Article
Citations
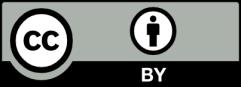
This work is licensed under a Creative Commons Attribution 4.0 International License , which permits unrestricted use, distribution, and reproduction in any medium, provided the original work is properly cited.