Open Access
ARTICLE
Tyre Inspection through Multi-State Convolutional Neural Networks
1 BMIE, Avinashilingam Institute for Home Science and Higher Education for Women, Coimbatore, 641043, India
2 Department of Computer Science and Engineering, Sahrdaya College of Engineering and Technology, Kerala, 680684, India
3 Department of Computer Science and Engineering, Anna University Regional Campus, Tirunelveli, 627007, India
4 School of Information Technology and Engineering, Vellore Institute of Technology, Vellore, 632014, India
5 Department of Information Technology, National Engineering College, Kovilpatti, 628503, India
6 Department of Mathematics and Computer Science, Faculty of Science, Beirut Arab University, 115020, Lebanon
7 Department of Computer Science and Engineering, Soonchunhyang University, Asan, 336745, Korea
* Corresponding Author: Yunyoung Nam. Email:
(This article belongs to the Special Issue: Recent Trends in Artificial Intelligence for Automated Complex Industrial Systems)
Intelligent Automation & Soft Computing 2021, 27(1), 1-13. https://doi.org/10.32604/iasc.2021.013705
Received 18 August 2020; Accepted 23 October 2020; Issue published 07 January 2021
Abstract
Road accident is a potential risk to the lives of both drivers and passers-by. Many road accidents occur due to the improper condition of the vehicle tyres after long term usage. Thus, tyres need to be inspected and analyzed while manufacturing to avoid serious road problems. However, tyre wear is a multifaceted happening. It normally needs the non-linearly on many limitations, like tyre formation and plan, vehicle category, conditions of the road. Yet, tyre wear has numerous profitable and environmental inferences particularly due to maintenance costs and traffic safety implications. Thus, the risk to calculate tyre wear is therefore of major importance to tyre producers, convoy owners and government. In this paper, we propose a Multi-state Convolution Neural Networks to analyze tyre tread patterns about wear and tear as well as tyre durability. The feature maps are identified from the input image through the Convolution functions that the sub-sampling utilizes for producing the output with the fully connected networks. The quadratic surface uses to perform the preprocessing of tyre images with several Convolutional layers. Through this work, we aim to reduce the economic implications as well as traffic safety implications which happen due to tyre wear. This will serve as a potential solution to tyre wear-related issues.Keywords
Cite This Article
C. Sivamani, M. Rajeswari, E. Golden Julie, Y. Harold Robinson, V. Shanmuganathan et al., "Tyre inspection through multi-state convolutional neural networks," Intelligent Automation & Soft Computing, vol. 27, no.1, pp. 1–13, 2021. https://doi.org/10.32604/iasc.2021.013705Citations
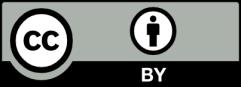