Open Access
ARTICLE
Experimental Evaluation of Clickbait Detection Using Machine Learning Models
1 Department of Computer Science and Information Technology, University of Engineering and Technology, Peshawar, Pakistan
2 University of Jeddah, College of Computer Science and Engineering, Department of Software Engineering, Jeddah, Saudi Arabia
3 University of Jeddah, College of Computing and Information Technology at Khulais, Department of Information Technology, Jeddah, Saudi Arabia
* Corresponding Author: Iftikhar Ahmad. Email:
Intelligent Automation & Soft Computing 2020, 26(6), 1335-1344. https://doi.org/10.32604/iasc.2020.013861
Received 24 August 2020; Accepted 25 September 2020; Issue published 24 December 2020
Abstract
The exponential growth of social media has been instrumental in directing the news outlets to rely on the stated platform for the dissemination of news stories. While social media has helped in the fast propagation of breaking news, it also has allowed many bad actors to exploit this medium for political and monetary purposes. With such an intention, tempting headlines, which are not aligned with the content, are being used to lure users to visit the websites that often post dodgy and unreliable information. This phenomenon is commonly known as clickbait. A number of machine learning techniques have been developed in the literature for automatic detection of clickbait. In this work, we consider six state of the art and classical machine learning algorithms, namely Support Vector Machine (SVM), Logistic Regression (LR), Naïve Bayes Classifier (NBC), Long Short Term Memory (LSTM), Parallel Convolutional Network (PNN), and Bidirectional Encoder Representations from Transformers (BERT) for automated clickbait detection. We also use four performance evaluation metrics, namely accuracy, precision, recall and F1-score to evaluate the performance of the selected set of machine learning algorithms on a real world data set. The results show that BERT is the best performing learning algorithm on three out of four evaluation metrics, and it achieves an average performance superiority of 3%–4% over all the other algorithms. Furthermore, it is observed that PNN has the worst performance among the selected algorithms.Keywords
Cite This Article
Citations
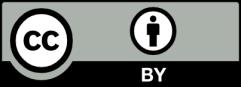
This work is licensed under a Creative Commons Attribution 4.0 International License , which permits unrestricted use, distribution, and reproduction in any medium, provided the original work is properly cited.