Open Access
ARTICLE
Soft Computing Based Evolutionary Multi-Label Classification
1 Department of Computer Science, COMSATS University Islamabad, Islamabad, 4550, Pakistan
2 Department of Computer Science & IT, The Islamia University of Bahawalpur, Bahawalpur, 63100, Pakistan
* Corresponding Author: Rubina Aslam. Email:
(This article belongs to the Special Issue: Soft Computing in Intrusion Detection)
Intelligent Automation & Soft Computing 2020, 26(6), 1233-1249. https://doi.org/10.32604/iasc.2020.013086
Received 25 July 2020; Accepted 28 August 2020; Issue published 24 December 2020
Abstract
Machine Learning (ML) has revolutionized intelligent systems that range from self-driving automobiles, search engines, business/market analysis, fraud detection, network intrusion investigation, and medical diagnosis. Classification lies at the core of Machine Learning and Multi-label Classification (MLC) is the closest to real-life problems related to heuristics. It is a type of classification problem where multiple labels or classes can be assigned to more than one instance simultaneously. The level of complexity in MLC is increased by factors such as data imbalance, high dimensionality, label correlations, and noise. Conventional MLC techniques such as ensembles-based approaches, Multi-label Stacking, Random k-label sets, and Hierarchy of Multi-label Classifiers struggle to handle these issues and suffer from the increased complexity introduced by these factors. The application of Soft Computing (SC) techniques in intelligent systems has provided a new paradigm for complex real-life problems. These techniques are more tolerant of the inherent imprecision and ambiguity in human thinking. Based on SC techniques such as evolutionary computing and genetic algorithms, intelligent classification systems can be developed that can recognize complex patterns even in noisy datasets otherwise invisible to conventional systems. This study uses an evolutionary approach to handle the MLC noise issue by proposing the Evolutionary Ensemble of Credal C4.5 (EECC). It uses the Credal C4.5 classifier which is based on imprecise probability theory for handling noisy datasets. It can perform effectively in diverse areas of multi-label classification. Experiments on different datasets show that EECC outperforms other techniques in the presence of noise and is noise-robust. Statistical tests show the significance of EECC as compared to other techniques.Keywords
Cite This Article
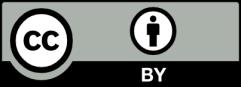
This work is licensed under a Creative Commons Attribution 4.0 International License , which permits unrestricted use, distribution, and reproduction in any medium, provided the original work is properly cited.