Open Access
ARTICLE
Text Detection and Classification from Low Quality Natural Images
1 Department of Computer Science, Wah Campus, COMSATS University Islamabad, Islamabad, 47040, Pakistan
2 Department of Computer Science, HITEC University, Taxila, 47080, Pakistan
3 Department of Mathematics and Computer Science, Beirut Arab University, Beirut, Lebanon
4 Department of Computer Science and Engineering, Soonchunhyang University, Asan, 31538, South Korea
* Corresponding Author: Yunyoung Nam. Email:
(This article belongs to the Special Issue: Recent Trends in Artificial Intelligence for Automated Complex Industrial Systems)
Intelligent Automation & Soft Computing 2020, 26(6), 1251-1266. https://doi.org/10.32604/iasc.2020.012775
Received 02 July 2020; Accepted 10 September 2020; Issue published 24 December 2020
Abstract
Detection of textual data from scene text images is a very thought-provoking issue in the field of computer graphics and visualization. This challenge is even more complicated when edge intelligent devices are involved in the process. The low-quality image having challenges such as blur, low resolution, and contrast make it more difficult for text detection and classification. Therefore, such exigent aspect is considered in the study. The technology proposed is comprised of three main contributions. (a) After synthetic blurring, the blurred image is preprocessed, and then the deblurring process is applied to recover the image. (b) Subsequently, the standard maximal stable extreme regions (MSER) technique is applied to localize and detect text. Soon after, K-Means is applied to get three different clusters of the query image to separate foreground and background and also incorporate character level grouping. (c) Finally, the segmented text is classified into textual and non-textual regions using a novel convolutional neural network (CNN) framework. The purpose of this task is to overcome the false positives. For evaluation of proposed technique, results are obtained on three mainstream datasets, including SVT, IIIT5K and ICDAR 2003. The achieved classification results of 90.3% for SVT dataset, 95.8% for IIIT5K dataset, and 94.0% for the ICDAR 2003 dataset, respectively. It shows the preeminence of the proposed methodology that it works fine for good model learning. Finally, the proposed methodology is compared with previous benchmark text-detection techniques to validate its contribution.Keywords
Cite This Article
Citations
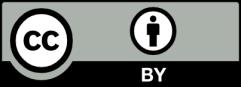