Open Access
ARTICLE
Deep 3D-Multiscale DenseNet for Hyperspectral Image Classification Based on Spatial-Spectral Information
1 School of Electronics and Information Engineering (School of Big Data Science), Taizhou University, Taizhou, 318000, China
2 College of Science, Heilongjiang Institute of Technology, Harbin, 150050, China
3 Departments of Interactive Technology, Animax Designs, Nashville, 37207, USA
* Corresponding Author: Weiwei Yang. Email:
Intelligent Automation & Soft Computing 2020, 26(6), 1441-1458. https://doi.org/10.32604/iasc.2020.011988
Received 09 June 2020; Accepted 07 July 2020; Issue published 24 December 2020
Abstract
There are two main problems that lead to unsatisfactory classification performance for hyperspectral remote sensing images (HSIs). One issue is that the HSI data used for training in deep learning is insufficient, therefore a deeper network is unfavorable for spatial-spectral feature extraction. The other problem is that as the depth of a deep neural network increases, the network becomes more prone to overfitting. To address these problems, a dual-channel 3D-Multiscale DenseNet (3DMSS) is proposed to boost the discriminative capability for HSI classification. The proposed model has several distinct advantages. First, the model consists of dual channels that can extract both spectral and spatial features, both of which are used in HSI classification. Therefore, the classification accuracy can be improved. Second, the 3D-Multiscale DenseNet is used to extract the spectral and spatial features which make full use of the HSI cube. The discriminant features for image classification are extracted and the spectral and spatial features are fused, which can alleviate the problem of low accuracy caused by limited training samples. Third, the connections between different layers are established using a residual dense block, and the feature maps of each layer are fully utilized to further alleviate the vanishing gradient problem. Qualitative classification experiments are reported that show the effectiveness of the proposed method. Compared with existing HSI classification techniques, the proposed method is highly suitable for HSI classification, especially for datasets with fewer training samples. The best overall accuracy of 99.36%, 99.86%, and 99.99% were obtained for the Indian Pines, KSC, and SA datasets, which showed an effective improvement of the classification accuracy.Keywords
Cite This Article
Citations
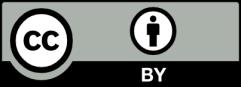
This work is licensed under a Creative Commons Attribution 4.0 International License , which permits unrestricted use, distribution, and reproduction in any medium, provided the original work is properly cited.