Open Access
ARTICLE
Multiple Faces Tracking Using Feature Fusion and Neural Network in Video
1 College of Mathematics and Econometrics, Hunan University, Changsha, 410082, China
2 College of Mathematics and Statistics, Hengyang Normal University, Hengyang, 421002, China
3 School of Computer Engineering and Applied Mathematics, Changsha University, Changsha, 410003, China
4 College of Computer Science and Electronic Engineering, Hunan University, Changsha, 410082, China
5 Key Laboratory of Digital Signal and Image Processing of Guangdong, Shantou, 515063, China
* Corresponding Author: Boxia Hu. Email:
Intelligent Automation & Soft Computing 2020, 26(6), 1549-1560. https://doi.org/10.32604/iasc.2020.011721
Received 26 May 2020; Accepted 07 August 2020; Issue published 24 December 2020
Abstract
Face tracking is one of the most challenging research topics in computer vision. This paper proposes a framework to track multiple faces in video sequences automatically and presents an improved method based on feature fusion and neural network for multiple faces tracking in a video. The proposed method mainly includes three steps. At first, it is face detection, where an existing method is used to detect the faces in the first frame. Second, faces tracking with feature fusion. Given a video that has multiple faces, at first, all faces in the first frame are detected correctly by using an existing method. Then the wavelet packet transform coefficients and color features from the detected faces are extracted. Furthermore, we design a backpropagation (BP) neural network for tracking the occasional faces. At last, a particle filter is used to track the faces. The main contributions are. Firstly, to improve face tracking accuracy, the Wavelet Packet Transform coefficients combined with traditional color features are utilized in the proposed method. It efficiently describes faces due to their discrimination and simplicity. Secondly, to solve the problem in occasional face tracking, and improved tracking method for robust occlusion tracking based on the BP neural network (PFT_WPT_BP) is proposed. Experimental results have been shown that our PFT_WPT_BP method can handle the occlusion effectively and achieve better performance over other methods.Keywords
Cite This Article
Citations
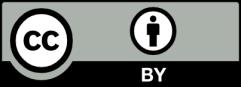
This work is licensed under a Creative Commons Attribution 4.0 International License , which permits unrestricted use, distribution, and reproduction in any medium, provided the original work is properly cited.