Open Access
ARTICLE
A PSO-XGBoost Model for Estimating Daily Reference Evapotranspiration in the Solar Greenhouse
1 National Engineering Research Center for Information Technology in Agriculture, Beijing, 100097, China
2 National Agro-tech Extension and Service Center, Beijing, 100125, China
3 School of Land Science and Technology, China University of Geosciences, Beijing, 100083, China
* Corresponding Author: Wengang Zheng. Email:
Intelligent Automation & Soft Computing 2020, 26(5), 989-1003. https://doi.org/10.32604/iasc.2020.010130
Abstract
Accurate estimation of reference evapotranspiration (ET0) is a critical prerequisite for the development of agricultural water management strategies. It is challenging to estimate the ET0 of a solar greenhouse because of its unique environmental variations. Based on the idea of ensemble learning, this paper proposed a novel ET0i estimation model named PSO-XGBoost, which took eXtreme Gradient Boosting (XGBoost) as the main regression model and used Particle Swarm Optimization (PSO) algorithm to optimize the parameters of XGBoost. Using the meteorological and soil moisture data during the two-crop planting process as the experimental data, and taking ET0i calculated based on the improved Penman–Monteith equation as the reference truth, the accuracy of model estimation was evaluated and the impact of less input variables on model estimation was tested. The results showed that PSO algorithm could optimize the parameters of XGBoost model stably, PSO-XGBoost model could accurately estimate ET0i in various data modes, and the estimation accuracy of the model decreases with the decrease of the number of input variables. Compared with other integrated learning models, PSO-XGBoost model could obtain the best estimation performance of ET0i.Keywords
Cite This Article
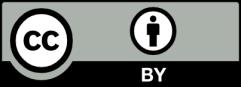
This work is licensed under a Creative Commons Attribution 4.0 International License , which permits unrestricted use, distribution, and reproduction in any medium, provided the original work is properly cited.