Open Access
ARTICLE
Reducing Operational Time Complexity of k-NN Algorithms Using Clustering in Wrist-Activity Recognition
Graduate School of Electrical and Computer Engineering, Ajou University
Suwon 443-749, Republic of Korea
* Corresponding Author: We-Duke Cho,
Intelligent Automation & Soft Computing 2020, 26(4), 679-691. https://doi.org/10.32604/iasc.2020.010102
Abstract
Recent research on activity recognition in wearable devices has identified a key challenge: k-nearest neighbors (k-NN) algorithms have a high operational time complexity. Thus, these algorithms are difficult to utilize in embedded wearable devices. Herein, we propose a method for reducing this complexity. We apply a clustering algorithm for learning data and assign labels to each cluster according to the maximum likelihood. Experimental results show that the proposed method achieves effective operational levels for implementation in embedded devices; however, the accuracy is slightly lower than that of a traditional k-NN algorithm. Additionally, our method provides the advantage of controlling the computational burden, depending on the performance of the embedded device on which the algorithm is implemented.Keywords
Cite This Article
Citations
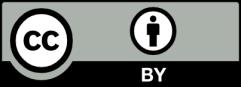
This work is licensed under a Creative Commons Attribution 4.0 International License , which permits unrestricted use, distribution, and reproduction in any medium, provided the original work is properly cited.