Open Access
ARTICLE
A Multi-objective Invasive Weed Optimization Method for Segmentation of Distress Images
1 Ph.D. candidate, Department of Building, Civil, and Environmental Engineering, Concordia University, Montreal, QC, Canada.
2 Assistant lecturer, Structural Engineering Department, Faculty of Engineering, Cairo University, Egypt.
3 Professor, Department of Building, Civil, and Environmental Engineering, Concordia University, Montreal, QC, Canada.
4 Professor, Structural Engineering Department, Faculty of Engineering, Cairo University, Egypt.
5 Professor, Department of Building and Real Estate, the Hong Kong Polytechnic University, Hung Hom, Hong Kong.
* Corresponding Author: Eslam Mohammed Abdelkader,
Intelligent Automation & Soft Computing 2020, 26(4), 643-661. https://doi.org/10.32604/iasc.2020.010100
Abstract
Image segmentation is one of the fundamental stages in computer vision applications. Several meta-heuristics have been applied to solve the segmentation problems by extending the Otsu and entropy functions. However, no single-objective function can optimally handle the diversity of information in images besides the multimodality issues of gray-level images. This paper presents a self-adaptive multi-objective optimization-based method for the detection of crack images in reinforced concrete bridges. The proposed method combines the flexibility of information theory functions in addition to the invasive weed optimization algorithm for bi-level thresholding. The capabilities of the proposed method are demonstrated through comparisons with singleobjective optimization-based methods, conventional segmentation methods, multi-objective genetic algorithm-based method, multi-objective particle swarmbased method and multi-objective harmony search-based method. The proposed method outperformed the previously-mentioned segmentation methods, whereas the average values of mean-squared error, peak signal to noise ratio and structural similarity index are equal to 0.0784, 11.4831 and 0.9921, respectively.Keywords
Cite This Article
Citations
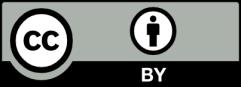
This work is licensed under a Creative Commons Attribution 4.0 International License , which permits unrestricted use, distribution, and reproduction in any medium, provided the original work is properly cited.