Open Access
ARTICLE
Wind Turbine Drivetrain Expert Fault Detection System: Multivariate Empirical Mode Decomposition based Multi-sensor Fusion with Bayesian Learning Classification
1 Research Scholar, Anna University, Assistant Professor, Department of ECE, Rajalakshmi Institute of Technology, Chennai, India
2 Professor, Velammal Engineering College, Chennai, India
* Corresponding Author: R.Uma Maheswari,
Intelligent Automation & Soft Computing 2020, 26(3), 479-488. https://doi.org/10.32604/iasc.2020.013924
Abstract
To enhance the predictive condition-based maintenance (CBMS), a reliable automatic Drivetrain fault detection technique based on vibration monitoring is proposed. Accelerometer sensors are mounted on a wind turbine drivetrain at different spatial locations to measure the vibration from multiple vibration sources. In this work, multi-channel signals are fused and monocomponent modes of oscillation are reconstructed by the Multivariate Empirical Mode Decomposition (MEMD) Technique. Noise assisted methodology is adapted to palliate the mixing of modes with common frequency scales. The instantaneous amplitude envelope and instantaneous frequency are estimated with the Hilbert transform. Low order and high order statistical moments, signal feature descriptors and randomness measures (entropy) are extracted as truthful features. The feature set is fed into the Bayes classifiers to compare the detection performance. From the analysis it is found that the proposed method is well performed with the Dynamic Bayes Belief Network Classifier showing the detection accuracy of 97.69%. To validate the results, the NRELWind Turbine Drivetrain benchmarking dataset is used.Keywords
Cite This Article
Citations
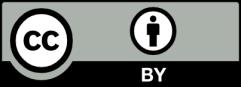