Open Access
ARTICLE
Extreme Learning Machine with Elastic Net Regularization
School of Electronic and information Engineering, South China University of Technology, Guangzhou, China, 510641.
* Corresponding Author: Lihua Guo,
Intelligent Automation & Soft Computing 2020, 26(3), 421-427. https://doi.org/10.32604/iasc.2020.013918
Abstract
Compared with deep neural learning, the extreme learning machine (ELM) can be quickly converged without iteratively tuning hidden nodes. Inspired by this merit, an extreme learning machine with elastic net regularization (ELM-EN) is proposed in this paper. The elastic net is a regularization method that combines LASSO and ridge penalties. This regularization can keep a balance between system stability and solution's sparsity. Moreover, an excellent optimization method, i.e., accelerated proximal gradient, is used to find the minimum of the system optimization function. Various datasets from UCI repository and two facial expression image datasets are used to validate the efficiency of our system. Final experimental results indicate that our ELM-EN requires less training time than multi-layer perceptron, and can achieve higher recognition accuracy than ELM and sparse ELM.Keywords
Cite This Article
Citations
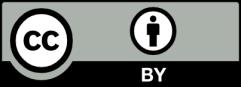
This work is licensed under a Creative Commons Attribution 4.0 International License , which permits unrestricted use, distribution, and reproduction in any medium, provided the original work is properly cited.