Open Access
ARTICLE
LSTM Neural Network for Beat Classification in ECG Identity Recognition
1 College of Communication Engineering, Jilin University, No. 2699, Qianjin Street, Changchun 130012, P. R. China
2 Zhuhai College of Jilin University, Jinwancaotang, Zhuhai 519041, P. R. China
* Corresponding Author: Xin Liu,
Intelligent Automation & Soft Computing 2020, 26(2), 341-351. https://doi.org/10.31209/2019.100000104
Abstract
As a biological signal existing in the human living body, the electrocardiogram (ECG) contains abundantly personal information and fulfils the basic characteristics of identity recognition. It has been widely used in the field of individual identification research in recent years. The common process of identity recognition includes three steps: ECG signals preprocessing, feature extraction and processing, beat classification recognition. However, the existing ECG classification models are sensitive to limitations of database type and extracted features dimension, which makes classification accuracy difficult to improve and cannot meet the needs of practical applications. To tackle the problem, this paper proposes to build an ECG individual recognition model based on a deep Long Short-Term Memory (LSTM) neural network. The LSTM network model has a memory cell and, therefore, it is an expert in handling long time ECG signals. With deeper learning, the nonlinear expression ability of the ECG beat classification model is gradually enhancing. The paper adopts two stacked LSTM models as hidden layers in the neural network; the Softmax layer is used as a classification layer to identify an individual. Then, low -level morphological features and deep-level chaotic features (Lyapunov exponent) are extracted to verify the feasibility of the deep LSTM network for classification. The model is respectively applied to a healthy human database and a human with a heart disease database. Experimental results show that extracting simple low-level features and chaotic features both achieve better classification performance. So, the robustness of the LSTM classification model is verified.Keywords
Cite This Article
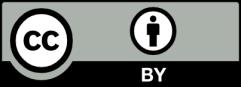
This work is licensed under a Creative Commons Attribution 4.0 International License , which permits unrestricted use, distribution, and reproduction in any medium, provided the original work is properly cited.