Open Access
ARTICLE
Self-Organizing Gaussian Mixture Map Based on Adaptive Recursive Bayesian Estimation
1 School of Finance, Zhejiang Gongshang University, Hangzhou, China
2 Hangzhou College of Commerce, Zhejiang Gongshang University, Hangzhou, Tonglu, China
* Corresponding Author: He Ni,
Intelligent Automation & Soft Computing 2020, 26(2), 227-236. https://doi.org/10.31209/2019.100000068
Abstract
The paper presents a probabilistic clustering approach based on self-organizing learning algorithm and recursive Bayesian estimation. The model is built upon the principle that the market data space is multimodal and can be described by a mixture of Gaussian distributions. The model parameters are approximated by a stochastic recursive Bayesian learning: searches for the maximum a posterior solution at each step, stochastically updates model parameters using a “dualneighbourhood” function with adaptive simulated annealing, and applies profile likelihood confidence interval to avoid prolonged learning. The proposed model is based on a number of pioneer works, such as Mixture Gaussian Autoregressive Model, Self-Organizing Mixture Map, and have some favoured attributes on its robust convergence and good generalization. The experimental results on both artificial and real market data show that the algorithm is a good alternative in measuring multimodal distribution.Keywords
Cite This Article
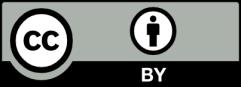
This work is licensed under a Creative Commons Attribution 4.0 International License , which permits unrestricted use, distribution, and reproduction in any medium, provided the original work is properly cited.