Open Access
ARTICLE
A Novel Knowledge-Based Battery Drain Reducer for Smart Meters
1 Department of Software Engineering. Mehran University of Engineering and Technology, Pakistan.
2 Department of Computer Science and Engineering, Hanyang University ERICA, 55 Hanyangdaehak-ro, Sangnok-gu, Ansan, Gyeonggi-do, 15588, Republic of Korea.
3 Department of Software Engineering, University of Lahore, Lahore, Pakistan.
* Corresponding Author: Scott Uk-Jin Lee,
Intelligent Automation & Soft Computing 2020, 26(1), 107-119. https://doi.org/10.31209/2019.100000132
Abstract
The issue of battery drainage in the gigantic smart meters network such as semantic-aware IoT-enabled smart meter has become a serious concern in the smart grid framework. The grid core migrates existing tabular datasets i.e., Relational data to semantic-aware tuples in its Resource Description Framework (RDF) format, for effective integration among multiple components to work aligned with IoT. For this purpose, WWW Consortium (W3C) recommends two specifications as mapping languages. However, both specifications use entire RDB schema to generate data transformation mapping patterns and results large quantity of unnecessary transformation. As a result, smart meters use huge computing resources, maximum energy capacity and come across battery drain problems. This paper proposes a novel semantic-aware battery drain optimization strategy ‘SPARQL Auto R2RML Mapping (SARM)’ that generates custom RDF patterns with precise metadata and avoids use of full schema along with optimized usage of network resources through (i) selective metadata migration, and (ii) optimal battery usage. The proposed approach effectively increases battery life with a balanced proportion of energy consumption and reduces meter load congestion which happens to be another vital reason of battery drain problem. The presented knowledge-based battery drain prevention strategy is evaluated over an RDB dataset using three types of SPARQL queries; Basic, Nested and Join. Furthermore, the R2RML processors evaluated SARM over the most recent Berlin SPARQL Benchmark datasets which depicts that SARM is efficient 40.4% in mapping generation time and 10.46% in average planning time than default RDB2RDF transformations. Finally, SARM significantly improves total execution time of RDB2RDF migration with an efficiency of 8.82% and conserves battery drain by 18.5% over the smart grid data cluster.Keywords
Cite This Article
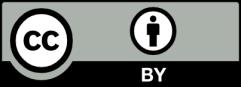
This work is licensed under a Creative Commons Attribution 4.0 International License , which permits unrestricted use, distribution, and reproduction in any medium, provided the original work is properly cited.