Open Access
ARTICLE
Image Classification Using Optimized MKL for SSPM
School of Information Engineering, Key Laboratory of Fiber Optic Sensing Technology and Information Processing, Wuhan University of Technology, Wuhan, Hubei, China
* Corresponding Author: Lu Wu,
Intelligent Automation & Soft Computing 2019, 25(2), 249-257. https://doi.org/10.31209/2018.100000010
Abstract
The scheme of spatial pyramid matching (SPM) causes feature ambiguity near dividing lines because it divides an image into different scales in a fixed manner. A new method called soft SPM (sSPM) is proposed in this paper to reduce feature ambiguity. First, an auxiliary area rotating around a dividing line in four orientations is used to correlate the feature relativity. Second, sSPM is performed to combine these four orientations to describe the image. Finally, an optimized multiple kernel learning (MKL) algorithm with three basic kernels for the support vector machine is applied. Specifically, for each level, a suitable kernel is selected to map the data that fall within the corresponding neighbourhood. In addition, a mixed-norm regularization formulation is optimized using MKL to solve the classification problem. The method proposed in this paper performs well when applied to the Caltech 101 and Scene 15 datasets. Experimental results are collected under various conditions. The results of sSPM are improved by nearly 4% compared with the existing experimental results.Keywords
Cite This Article
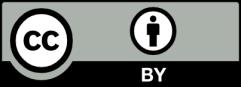