Open Access
ARTICLE
The Design and Implementation of a Multidimensional and Hierarchical Web Anomaly Detection System
* P.O. Box 202, Beijing University of Posts and Telecommunications, Haidian District, Beijing, 100876, China.
State Key Laboratory of Networking and Switching Technology
Beijing University of Posts Telecommunications, Beijing, 100876, China
jfguan@bupt.edu.cn, ljwemls@gmail.com, zbjiang@bupt.edu.cn
* Corresponding Author: Jianfeng Guan,
Intelligent Automation & Soft Computing 2019, 25(1), 131-141. https://doi.org/10.31209/2018.100000050
Abstract
The traditional web anomaly detection systems face the challenges derived from the constantly evolving of the web malicious attacks, which therefore result in high false positive rate, poor adaptability, easy over-fitting, and high time complexity. Due to these limitations, we need a new anomaly detection system to satisfy the requirements of enterprise-level anomaly detection. There are lots of anomaly detection systems designed for different application domains. However, as for web anomaly detection, it has to describe the network accessing behaviours characters from as many dimensions as possible to improve the performance. In this paper we design and implement a Multidimensional and Hierarchical Web Anomaly Detection System (MHWADS) with the objectives to provide high performance, low latency, multi-dimension and adaptability. MHWADS calculates the statistical characteristics, and constructs the corresponding statistical model, detects the behaviour characteristics to generate the multidimensional correlation eigenvectors, and adopts several classifications to build an ensemble model. The system performance is evaluated based on realistic dataset, and the experimental results show that MHWADS yields substantial improvements than the previous single model. More important, by using 2-fold Stacking as the ensemble architecture, the detection precision and recall are 0.99988 and 0.99647, respectively.Keywords
Cite This Article
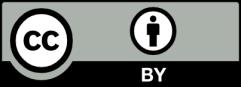