Open Access
ARTICLE
A Novel Strategy for Mining Highly Imbalanced Data in Credit Card Transactions
Institute of Image Processing and Pattern Recognition, Shanghai Jiao Tong University, Shanghai, China
* Corresponding Author: Jie Yang,
Intelligent Automation & Soft Computing 2018, 24(4), 721-727. https://doi.org/10.1080/10798587.2017.1321228
Abstract
The design of an efficient credit card fraud detection technique is, however, particularly challenging, due to the most striking characteristics which are; imbalancedness and non-stationary environment of the data. These issues in credit card datasets limit the machine learning algorithm to show a good performance in detecting the frauds. The research in the area of credit card fraud detection focused on detection the fraudulent transaction by analysis of normality and abnormality concepts. Balancing strategy which is designed in this paper can facilitate classification and retrieval problems in this domain. In this paper, we consider the classification problem in supervised learning scenario by creating a contrast vector for each customer based on its historical behaviors. The performance evaluation of proposed model is made possible by a real credit card data-set provided by FICO, and it is found that the proposed model has significant performance than other state-of-the-art classifiers.Keywords
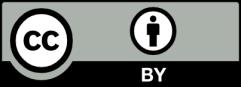